Curtis Bostick
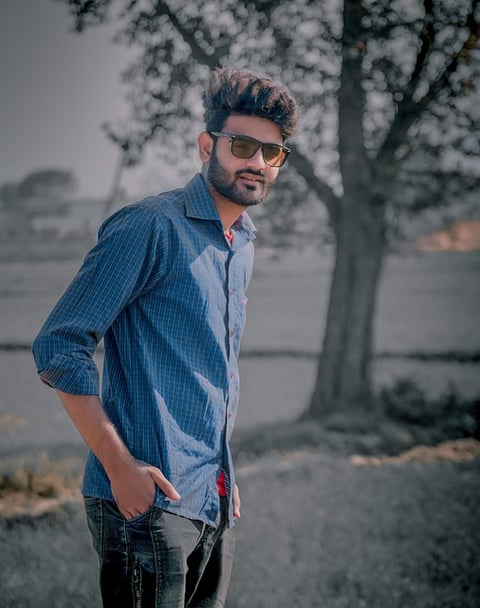
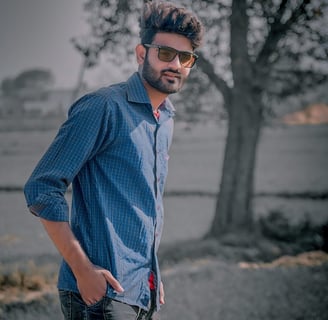
Professional Summary:
Curtis Bostick is a visionary astrophysicist and computational cosmologist, specializing in the development of generative models to simulate and analyze the large-scale structure of the universe. With a deep understanding of cosmology, machine learning, and high-performance computing, Curtis is dedicated to unraveling the mysteries of cosmic evolution by creating sophisticated models that replicate the distribution of galaxies, dark matter, and cosmic voids. His work bridges the gap between theoretical cosmology and observational data, providing critical insights into the universe’s formation and growth.
Key Competencies:
Generative Model Development:
Designs advanced generative models, such as deep learning architectures and probabilistic frameworks, to simulate the large-scale structure of the universe.
Ensures that models accurately capture the statistical properties and physical processes of cosmic evolution.
Cosmological Data Analysis:
Analyzes large-scale structure data from surveys like the Sloan Digital Sky Survey (SDSS) and the Euclid Space Telescope to validate and refine generative models.
Develops pipelines to compare model predictions with observational data, identifying discrepancies and improving model fidelity.
High-Performance Computing:
Leverages supercomputing resources to scale generative models, enabling simulations of vast cosmic volumes with high resolution.
Optimizes algorithms for efficiency, reducing computational costs while maintaining accuracy.
Interdisciplinary Collaboration:
Collaborates with cosmologists, data scientists, and machine learning experts to integrate diverse perspectives into model development.
Contributes to international research initiatives focused on understanding the universe’s large-scale structure.
Research & Innovation:
Publishes groundbreaking research on generative models for cosmology in leading astrophysics and machine learning journals.
Explores emerging technologies, such as quantum computing and generative adversarial networks (GANs), to push the boundaries of cosmic simulations.
Career Highlights:
Developed a generative model that accurately replicated the cosmic web, providing new insights into the distribution of dark matter and galaxies.
Contributed to the analysis of SDSS data, identifying patterns in the large-scale structure that supported key cosmological theories.
Published influential research on generative models for cosmology, earning recognition at international astrophysics and AI conferences.
Personal Statement:
"I am driven by a passion for understanding the universe’s large-scale structure and a commitment to developing generative models that bridge the gap between theory and observation. My mission is to create simulations that not only replicate the cosmos but also reveal its deepest secrets."
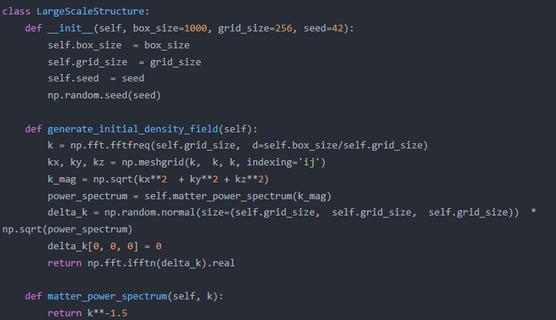
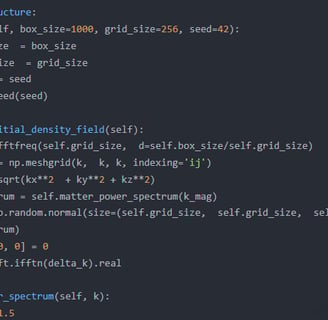
Data Validation
Comparing model outputs with observational data to ensure accuracy and reliability.
Parameter Exploration
Using models to investigate the effects of cosmological parameters on large-scale structure evolution.
Tobetterunderstandthecontextofthissubmission,IrecommendreviewingmypreviousworkontheapplicationofAIincosmology,particularlythestudytitled"EnhancingCosmologicalSimulationsUsingAIDrivenGenerativeModels."Thisresearchexploredtheuseofmachinelearningandoptimizationalgorithmsforimprovingthequalityandrelevanceofcosmologicalsimulations.Additionally,mypaper"AdaptingLargeLanguageModelsforDomainpecificApplicationsinCosmologicalAI"providesinsightsintothefinetuningprocessanditspotentialtoenhancemodelperformanceinspecializedfields.